Blog post
Loosely inspired by the brain
Neural networks are commonly characterized as being “loosely being inspired by the brain”, but what does that really mean and why does it matter?
Does the future of machine intelligence depend on what we understand about
the brain?
Despite critical advancements in machine learning – notably the rise of GenAI – lessons from the human brain remain. The way humans’ learn continues to be both the inspiration and the persistent benchmark for many types of AI system.

Brain and machines: beyond metaphor
The remarkably convincing human-like responses generated by ChatGPT and other large language model (LLM) chatbots, has reignited popular discourse on intelligent machines. AI related news has surged as companies scramble to invest in Generative AI (GenAI) and growing ethical and legal concerns come to the fore. Wider public narratives about artificial brains displacing their biological counterparts raises the question – what do deep neural networks really have in common with the human brain? Deep neural networks – the current dominant form of AI – are often characterized as being loosely inspired by the brain. Inspired by the functioning of biological neurons, knowledge in artificial neural networks is represented through “patterns of activation”, created through varying strengths in connections between artificial ‘neurons’. Human cognition is itself characterized by elementary processing units which form dense connections in the brain, enabling us to solve a myriad of complex tasks, generate novel strategies and abstract knowledge from limited examples.
The field of computer science is no stranger to borrowing concepts from human cognition. For instance, the brain as a metaphor for Computer Processing Unit (CPU) is often used to illustrate its role as an information and processing hub in modern computing architectures. However, the relationship between the brain and AI goes far beyond metaphor and analogy. The specific structure and design of an AI/machine learning system dictates fundamental assumptions about the way information is distributed and represented. For a system to engage learning mechanisms, it needs a way to represent, store and access information. For instance, deep neural networks can be categorized as sub-symbolic systems – information is represented through parallel distributions of the input data’s statistical properties. Framing innovations in deep learning as advancements in machine intelligence requires some conception of how the underlying computations may relate to cognitive capabilities.
A defining factor of the successes of transformer-based language models is scale – more parameters and more data. This not-so-secret ingredient has spurred on the development of larger and larger models. While the architectural innovations that led up to the development of large language models (LLMs) should not be reduced to ‘throwing data at the problem’, the reliance on scale is a key issue for the future of language models. The pursuit of larger and larger models is exacerbating issues around environmental and financial sustainability as well as highlighting the need for greater governance and documentation of large corpuses of unlabeled data sourced from the public internet. The reliance on scale for performance also calls into question the viability of LLMs as the future of intelligent machines. ‘Grand unified theories’ of intelligence may not be necessary for the evolution of AI or indeed be achievable. However, the current big questions in AI may signal the need to reexamine the potential role of cognitive theory.
The field of artificial intelligence began its roots in cognitive science - modern deep learning architectures are grounded in neuroscience and psychology. Indeed, the initial conception of artificial neurons was based on pioneering work by the neurophysiologist Charles Scott Sherrington and the neuroscientist Santiago Ramón y Cajal. Warren McCulloch and Walter Pitts’ 1943 computational model of the neuron formed the basis of the modern neural network. Better known as the McCulloch-Pitts computational neuron, this early artificial neuron modelled neural firing and spiking with threshold activation functions. Donald Hebb’s workm published in 1949, outlined how neural pathways strengthen through use – ‘cells that fire together, wire together’ – informed the development of the Perceptron. Unlike the McCulloch-Pitts neuron, the Perceptron went beyond summing the weights of neurons and used a learning mechanism to vary the weights of different inputs.
Concepts inspired by human cognition, like reinforcement learning and ‘self-attention’ – a critical mechanism in the Transformer architecture – are fundamental features of modern deep learning. However, despite the impressive results of Transformer based LLMs, they depend on a massive amount of linguistic context, are unable to intuit their own knowledge and are computationally and energy intensive. Human cognition, in comparison, isn't energy intensive or dependent on extensive linguistic contexts. The successes of machine learning engineering has somewhat obscured the role of scientific theory in the quest to define and reproduce intelligence. As current instantiations of deep learning frameworks are proving to be effective, the field has less motivation to stay faithful to a combined project of intelligence – scientific theory unifying both machine and human intelligence research. Staying faithful to theory for the theory’s sake is not the path to innovation. However, the limitations of current LLMs highlight the lessons that are still to be learnt from cognitive theory as the field of artificial intelligence continues to develop. Indeed, the engineering successes of particular deep neural network architectures and model development cycles should not discourage the exploration and development of scientific theory. Both engineering and theory have a vital role to play when shaping and defining machine intelligence. ‘It is interesting to try clarify what artificial intelligence is still missing, because this is also a way to identify what is unique about our species’ learning abilities’ - Stanislas Dehaene, How we Learn.
The problem of intelligence: a multidisciplinary project
The quest for intelligence has historically been a multi-disciplinary project comprised of neuroscience, psychology, cognitive science and computer science. Indeed, the development and evolution of AI is a story closely intertwined with research into human intelligence. However, as the pace of machine learning research continues to accelerate in the wake of the hype surrounding GenAI, the problem of intelligence is no longer the combined project it once was. Although humans continue to be the persistent benchmark in measuring the performance and effectiveness of AI, the focus on uncovering the mysteries of the human brain as a key to machine intelligence has somewhat faded. High-profile commercial machine learning applications are not new, however the recent highly publicized successes of OpenAI’s GPT has led some commentators to suggest the problem of intelligence, if it isn’t already, is on the verge of being solved.
While the expressive and coherent output generated by ChatGPT is certainly temptingly compelling evidence, the conversational nature of its output also suggests its limitations – its reliance on a massive training corpus. The exact training resources used by OpenAI for later versions of GPT are not publicly available. However, in addition to Wikipedia and other online resources, it appears popular LLMs may also be trained on thousands of pirated fiction and non-fiction books. Current LLMs predict one word at a time, based on the context of its already generated text. However, humans notably generate language and text in complete expressions and phrases. Ev Fedorenko, an associate professor of brain and cognitive sciences, highlights that unlike humans, LLMs’ rely on long linguistic contexts for language processing. Alison Gopik, a professor of psychology commented that ‘large language models are valuable cultural technologies that can imitate millions of human writers’, ‘but ultimately, machines may need more than large scale language and images to match the achievements of every human child.’
The substantial amounts of data required to train supervised/semi-supervised machines is a far cry from the brain’s ability to efficiently learn. The capacity of human children to rapidly learn, generalize and identify categories from limited examples is frequently cited as an indication of the uniqueness of human intelligence. In addition to the sheer quantity of data required to train neural networks, the training and deployment process generates high energy costs. The interconnectivity of the brain is, however, far more efficient. As the neuroscientist Stanislas Dahaene states, human learning is exceptional because it is flexible, it can operate on scarce data and because we can generalize, abstract knowledge and apply it to different situations. The neuroscientist Jeff Hawkin’s company Numenta seeks to reduce neural network’s carbon footprint by taking inspiration from the brain. One technique includes ‘sparsifying’ the network, a process which involves constraining the activation and connectivity (the weights) of the network to make it smaller and more efficient. Although the initial training compute intensive stage is not circumvented by this technique, it may provide insight into how sparsity can support better model generalization. Other engineering techniques like quantization and sharding developed to support memory constraints, represent engineering innovations that improve the practical implementations of large models. However, to tackle the fundamental research issues at hand – including the environment and financial costs of increasingly large models, frameworks based on theory are critical.
For some who study intelligence, neural networks are brute force statistical machines that lack the higher lever reasoning and logical processes characterized by the symbolic systems which were popular in the 1980s. Hardware optimization, transfer learning, sparsifying and other engineering techniques inspired by the brain may improve neural networks efficiency and performance across other metrics. However, deep neural networks may not be the only AI paradigm that can support intelligence in machines. Integration with rule-based systems could be a critical factor in the development of future AI systems. The computations supported by artificial neural networks may only be analogous to a certain type of brain computation and other types of systems may be necessary to form abstract knowledge and symbolic representations.
How do brains and machines see?
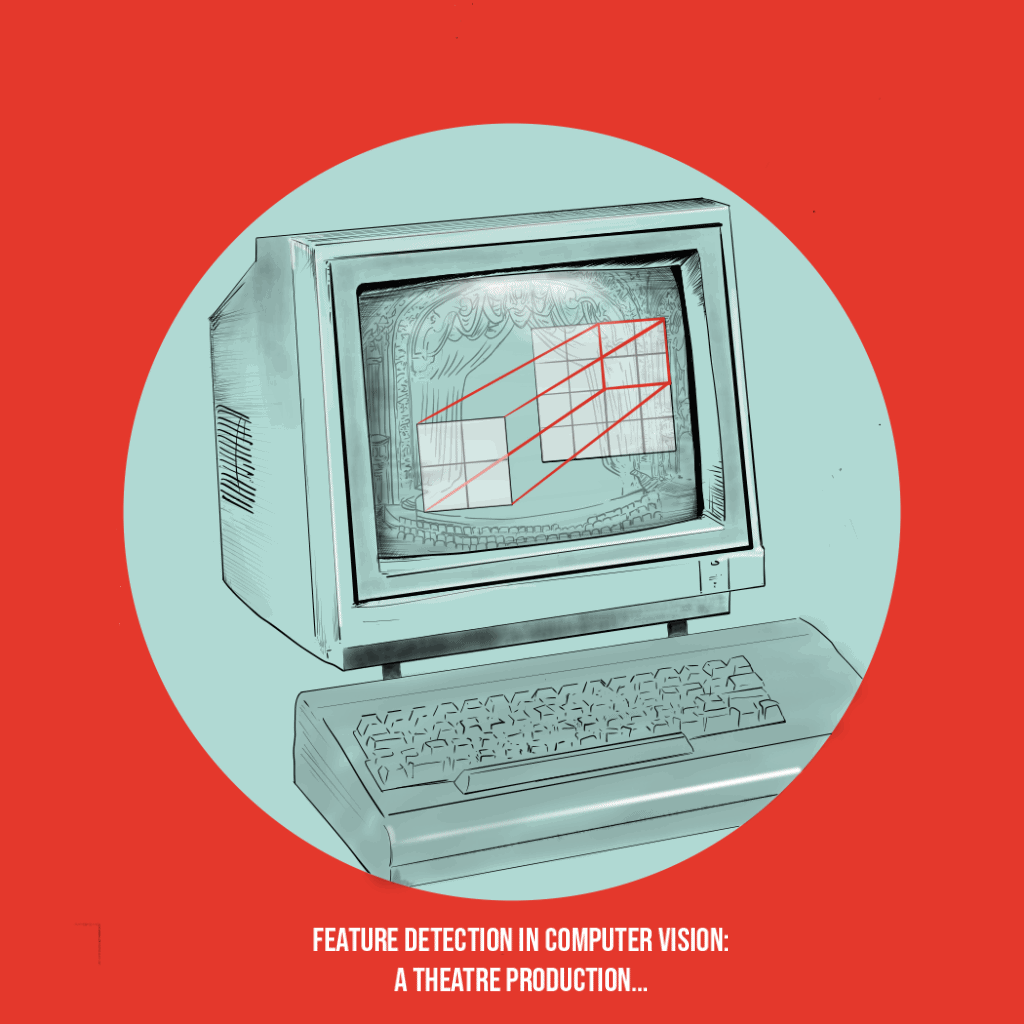
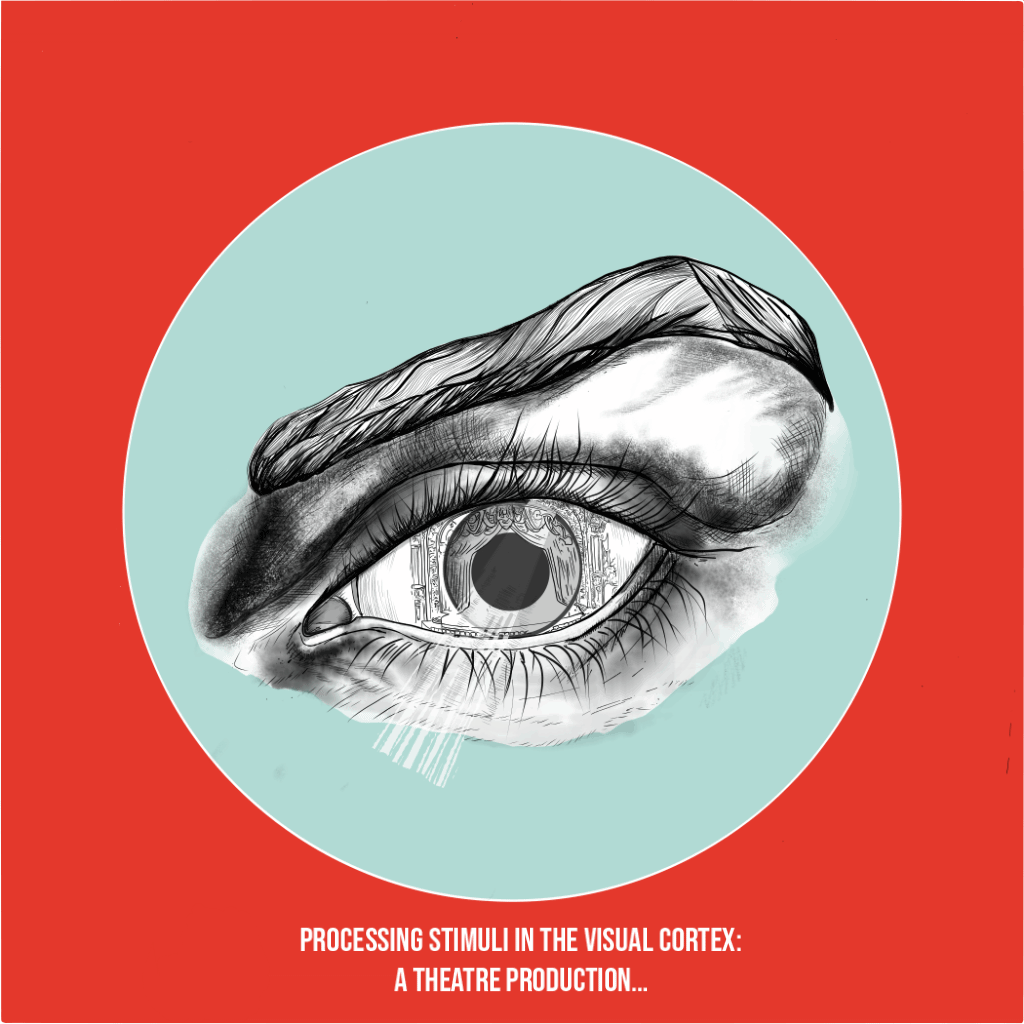
Before the highly publicized success of LLMS, computer vision could be considered the fashionable field in AI as it experienced a lot of progress. Computer vision models – supported by the Convolutional Neural Network architecture – now rival humans when it comes to visual recognition tasks. As well as being highly effective, the similarities between CNNs and the visual ventral pathway in primates can also provide an intuitive example of how the mechanisms and functioning of the brain can inspire artificial neural networks. The hierarchical processing of the visual ventral pathway, revealed in Hubel and Weisel’s seminal 1962 experiment with cats’ eyes, is analogous to the series of stacked layers that form modern neural networks. Hubel and Weisel’s work linked the retina to the cortex and exposed the orientation selectivity of neurons in the V1 region of the visual cortex.
Since Hubel and Weisel’s study, a lot of research has been conducted to investigate the theory that the ventral visual stream hierarchically processes increasingly complex feature representations, a process that peaks in feature complexity at the inferior temporal cortex. In the case of CNNs, feature maps are created through convolutions and sets of filters, which are activated by different features of varying levels of complexity. The neural responses to retinal information in the V1 are relatively well-understood in comparison to “higher ventral areas”, which are thought to support more sophisticated visual object recognition. Later layers in neural networks are also able to selectively process specific objects while becoming more tolerant to natural variability and the noise in images. Yamins et al., note that deeper CNN networks “correspond intuitively to architecturally specialized subregions like those observed in the ventral visual stream”.
Cognitive science theory does not need to be an abstract and esoteric part of contemporary machine learning. If human capabilities continue to be the persistent benchmark for AI systems, should they not also continue to be their inspiration?
The pandemonium architecture
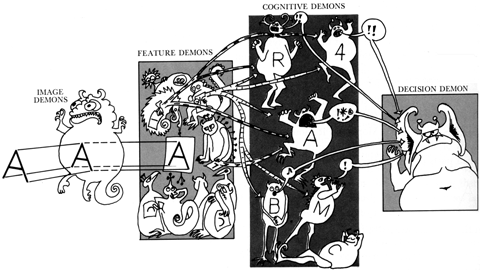
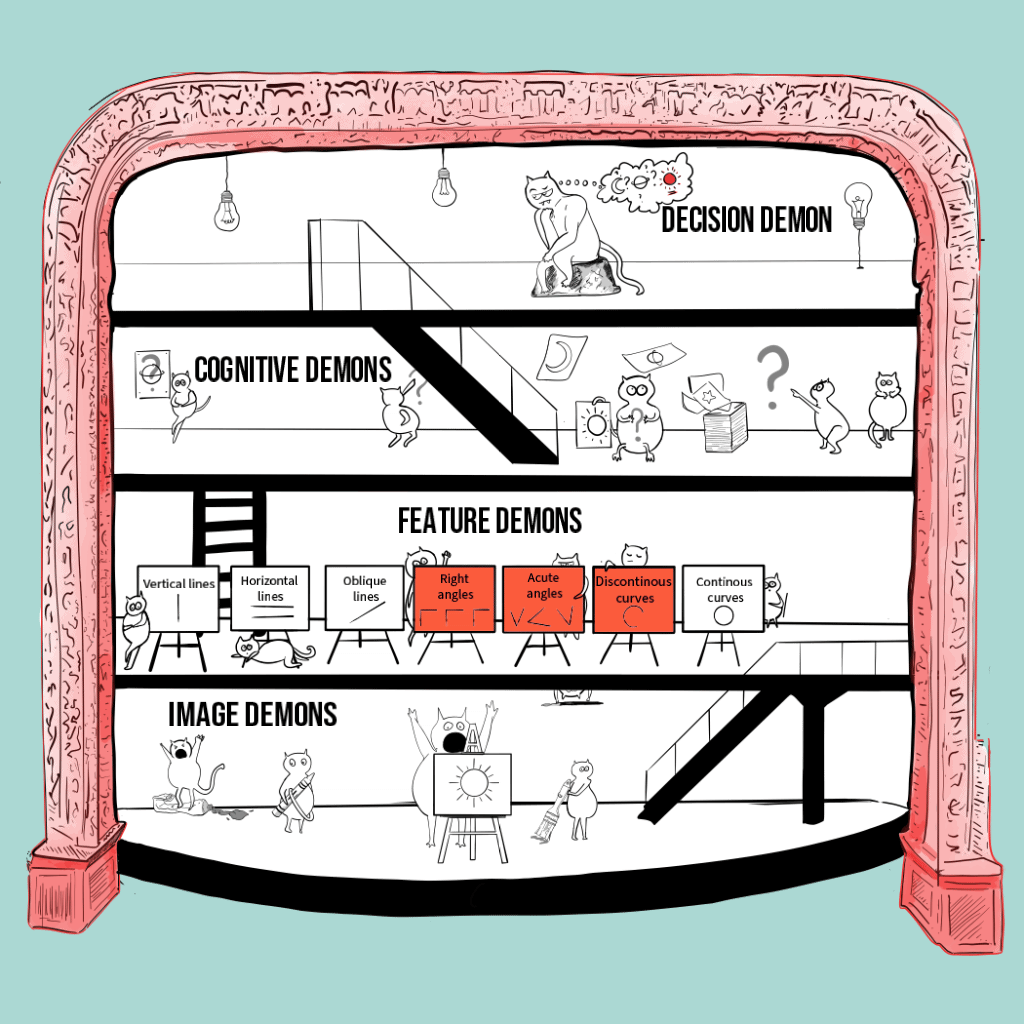
Image on the right: My interpretation of the pandemonium architecture